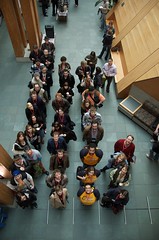
Revise/Remix can build a 1000 points of OER light. Can these lights converge to fire authentic learning analytics & share-alike data models?
Conventional wisdom in the OER community maintains that one of the more important features of the open education approach is the malleability and customizability of materials, allowing freely available component resources to be remixed, adapted and modified to suit specific institutional directives, student needs or faculty interests. These features are important enough that the ability to revise and remix content is a core part of the commonly accepted 4R framework that defines open content. While the ability to tailor OER to meet changing or specific needs is one compelling part of the open model, the infinity variety that this encourages creates serious obstacles for another expected benefit of openness: using learning analytics to drive adaptive teaching and learning, support iterative improvement, and demonstrate effectiveness.
The ability to deliver meaningful learning analytics has been one promise of the open education approach. A use-driven design process for OER depends on the resources being used by a large number of students with varied background knowledge, relevant skills and future goals—a student population that open and well-used resources should be able to provide. Such a process can use such interaction data to iteratively improve courses in a meaningful and empirical way. Beyond improvement analytics, this same data can be used mid-stream to improve the effectiveness of learners and instructors.
Despite this promise, the OER community has not been able to create or take advantage of widespread, generally applicable learning analytics tools. While some organizations have had success in developing analytics platforms and approaches, such successes have tended to focus on specific resources, often developed with data collection in mind and not always falling at the “most open” end of the open content continuum. One barrier to more widespread analytic tools has been the variety of OER afforded by remixing and revising.
This presentation will explore the benefits and trade-offs to be made between adaptability and analytics. In the course of this exploration, we will argue that the benefits to be had from an approach that places a higher priority on analytics may outweigh those to be gained from endless variety in the OER space. Similarly, we will discuss some approaches to better harness open education’s promised ability to drive learning analytics, with greater and lesser compromises to the adaptability of OER. We will propose open communities of use and evaluation coalesced around individual OERs using learning analytics to improve the resource through coordinated revision and remix. Open education has embraced share alike licenses for materials. The next logical step is the open exchange of learning data and evidence of effectiveness, to “share alike and share data”. We will also suggest approaches to integrating disparate analytics-enabled OER into common platforms and the development of OER to published standards for learning analytic data.
Notes:
Open Learning Initiative:
Produce and improve scientifically-based courses and course materials which enact instruction and support instructors
Outcomes:
Shared understanding of challenges, tensions, and possibilities in learning analytics
Describe community-based analytics plans
Driving feedback loops. We have a huge opportunity to use assessment data around OERs. There are enormous amounts of data available. "Infinite points of light" around all the OER repositories and initiatives.
Infinite proliferation
The 4 Rs
- Reuse
- Redistribute
- Revising
- Remix
Not recreate but to evaluate - recreate is a barrier to reuse.
What drives change in these settings?
- Data
- Intuition
- Market demand
- Instructor preferences - change happens because an instructor gets bored with material, but with no real idea if the changes are improving the course.
Effectiveness: An OER is effective when it demonstrably supports students in meeting articulated, measurable learning outcomes in a given set of contexts.
They are also looking at new forms of assessment for gathering data.
Rory McGreal asked whether or not there was a clearer way to talk about what is demonstrable. There are a lot of variables in the initial statement.
Why have we not been doing this?
- It's hard
- Costly
- Individual faculty need support
- It can be threatening to educators
- Disparate systems for collecting data
What do we mean by "learning analytics"?
Proxies vs. authentic assessment and evaluation
Analytics Definition
Data collection > reporting > Decision making > Intervention > Action
Collecting data is not enough. We also need to make sense of it in ways that are actionable.
Types of analytics
Education/classroom management
Learning outcomes
We need common, agreed upon standards, a core collection, and a space for exploration.
Problems include privacy and technical issues.
Tools that already exist:
DataShop, Evidence Hub, Learning registry, and communities of evidence
Community College Open Learning Initiative
And build new things:
We need better mechanisms to share data.
We need a community-based approach.
Learning Intelligence Systems
What would be giving up? This approach forces us to allow our minds to be charged by evidence.
Next Steps?
Innovate
Standardize
Scale
- Commitment to assessment and evaluation
- Community definition of analytics enabled OER
- Common approach to data
- Shared and private analytics platforms
No comments:
Post a Comment